Researchers have predicted the outcome after simulating the entire soccer tournament 100,000 times.
The 2018 soccer World Cup kicks off in Russia on Thursday and is likely to be one of the most widely viewed sporting events in history, more popular even than the Olympics. So the potential winners are of significant interest.
One way to gauge likely outcomes is to look at bookmakers’ odds. These companies use professional statisticians to analyze extensive databases of results in a way that quantifies the probability of different outcomes of any possible match. In this way, bookmakers can offer odds on all the games that will kick off in the next few weeks, as well as odds on potential winners.
An even better estimate comes from combing the odds from lots of different bookmakers. This approach suggests Brazil is the clear favorite to win the 2018 World Cup, with a probability of 16.6 percent, followed by Germany (12.8 percent) and Spain (12.5 percent).
But in recent years, researchers have developed machine-learning techniques that have the potential to outperform conventional statistical approaches. What do these new techniques predict as the likely outcome of the 2018 World Cup? First some background. The random-forest technique has emerged in recent years as a powerful way to analyze large data sets while avoiding some of the pitfalls of other data-mining methods. It is based on the idea that some future event can be determined by a decision tree in which an outcome is calculated at each branch by reference to a set of training data.An answer comes from the work of Andreas Groll at the Technical University of Dortmund in Germany and a few colleagues. These guys use a combination of machine learning and conventional statistics, a method called a random-forest approach, to identify a different most likely winner.
Recommended for You
However, decision trees suffer from a well-known problem. In the latter stages of the branching process, decisions can become severely distorted by training data that is sparse and prone to huge variation at this kind of resolution, a problem known as overfitting.
The random-forest approach is different. Instead of calculating the outcome at every branch, the process calculates the outcome of random branches. And it does this many times, each time with a different set of randomly selected branches. The final result is the average of all these randomly constructed decision trees.
This approach has significant advantages. First, it does not suffer from the same overfitting problem that plagues ordinary decision trees. It also reveals which factors are most important in determining the outcome.
So if a particular decision tree includes lots of parameters, it becomes easy to see which ones have the biggest impact on the outcome and which do not. These less important factors can then be ignored in future.
Groll and co use exactly this approach to model the 2018 World Cup. They model the outcome of each game the teams are likely to play and use the results to construct the most probable course of the tournament.
Groll and co begin with a wide range of potential factors that might determine the outcome. These include economic factors such as a country’s GDP and population, FIFA’s ranking of national teams, and the properties of the teams themselves, such as their average age, the number of Champions League players they have, whether they have home advantage, and so on.
Interestingly, the random-forest approach allows Groll and co to include other ranking attempts, such as the rankings used by bookmakers.
Plugging all this into the model provides some interesting insights. For example, the most influential factors turn out to be the team rankings created by other methods, including those from bookmakers, FIFA, and others.
Other important factors include GDP and the number of Champions League players on the team. Unimportant factors include the country’s population, the nationality of the coach, and so on.
The predictions arrived at through this process differ from others in some important ways. For a start, the random-forest method picks out Spain as the most likely winner, with a probability of 17.8 percent.
However, a big factor in this prediction is the structure of the tournament itself. If Germany clears the group phase of the competition, it is more likely to face strong opposition in the 16-team knockout phase. Because of this, the random-forest method calculates Germany’s chances of reaching the quarter-finals as 58 percent. By contrast, Spain is unlikely to face strong opposition in the final 16 and so has a 73 percent chance of reaching the quarter-finals.
If both make the quarter-finals, they have a more or less equal chance of winning. “Spain is slightly favored over Germany mainly due to the fact that Germany has a comparatively high chance to drop out in the round-of-sixteen,” say Groll and co.
But there is an additional twist. The random-tree process makes it possible to simulate the entire tournament, and this produces a different result.
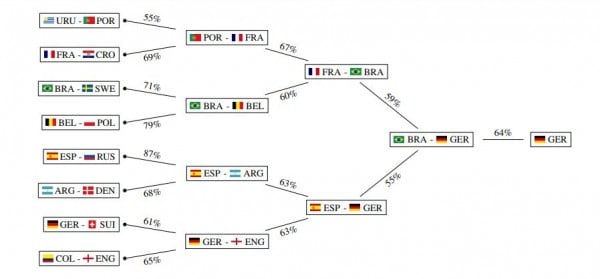
Groll and co simulated the entire tournament 100,000 times. “According to the most probable tournament course, instead of the Spanish the German team would win the World Cup,” they say.
Of course, because of the huge number of permutations of games, this course is still extremely unlikely. Groll and co put the odds at about 1 in 100,000.
So there you have it. At the beginning of the tournament, Spain has the best chances of winning, according to Groll and co. But if Germany makes the quarter-finals, it then becomes the front-runner.
The tournament kicks off on Thursday, when the hosts, Russia, take on Saudi Arabia. Sadly, neither of these teams looks likely to make even the quarter-finals.
Ref: arxiv.org/abs/1806.03208 : Prediction Of The FIFA World Cup 2018 – A Random Forest Approach With An Emphasis On Estimated Team Ability Parameters